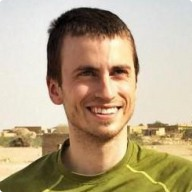
Accelerate your Maven CI builds with distributed named locks using Redis
Everybody loves a fast, responsive continuous integration (CI) setup. While it’s fun to battle each others on rolling chairs, having quick feedback on pull requests builds and fast deployments is an important part of a good development environment and is also something we constantly have to invest time in.
In this post, we will show you a neat way to safely use your local Maven repository across multiple build processes using the Named Locks feature of Maven Artifact Resolver. This will speed up your concurrent builds by reducing the required time to download dependencies and will also help maximize your CI instances usage.
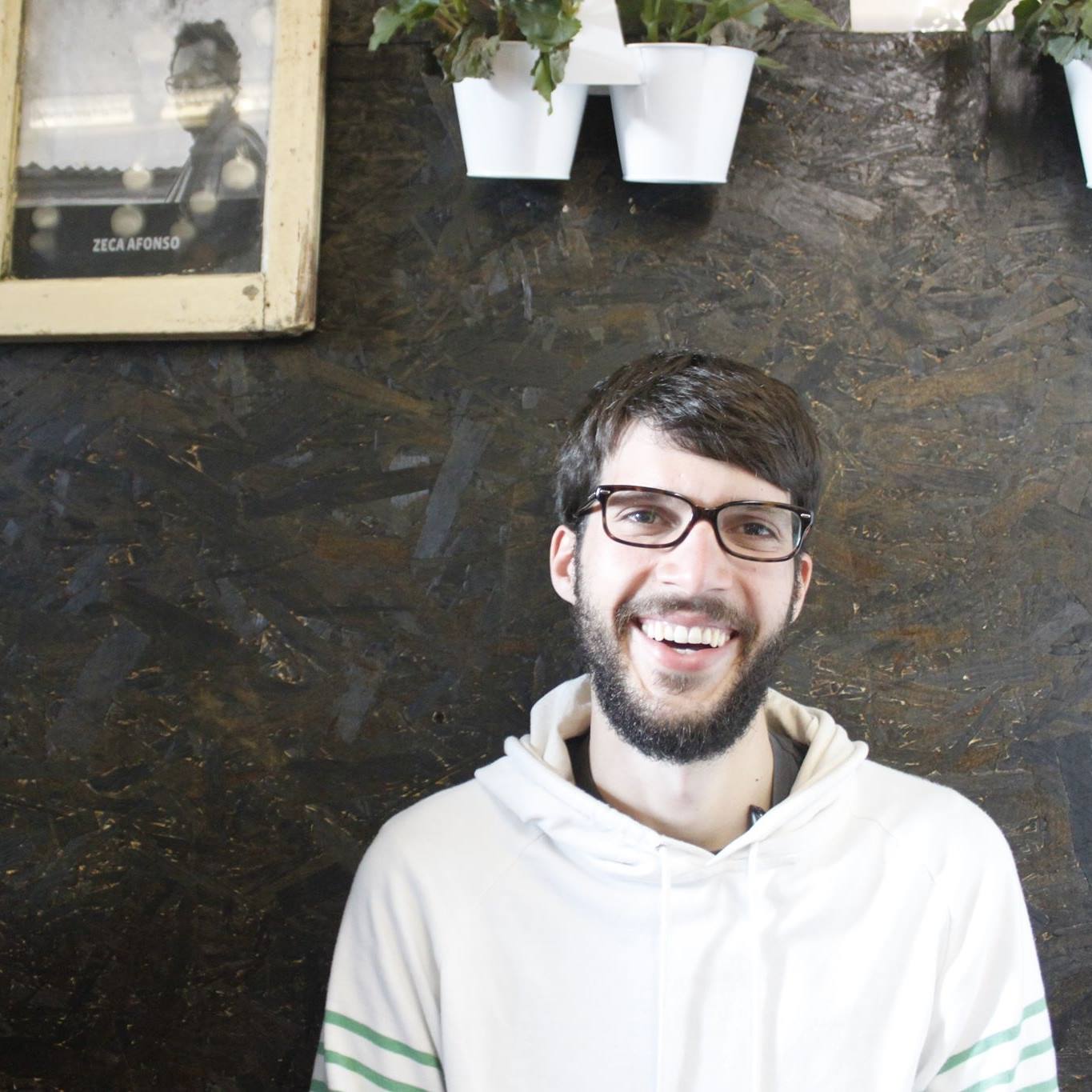
Improving download speed of a S3 proxy in Go
There is an old adage that says, “Hardware eventually fails. Software eventually works.” As the Coveo Blitz competition is approaching, we are ramping up the platform to receive more than 135 participants who will produce software bots that fight against one another for the prestigious Coveo Cup. One of the cool features of the platform, that we’ve created and improved over the years, is the ability to visualize past fights by downloading a replay file.
But this year, like every year, was different. This year, the replays were up to 50MiB large and the download time was suffering from it. By suffering I mean 50 seconds to download 40MiB; waiting for a mp3 to download in the 2000’s kind of suffering. The replays are stored in S3, proxied by our servers written in go, so let’s see what is going on in the go code, and maybe it will eventually work!
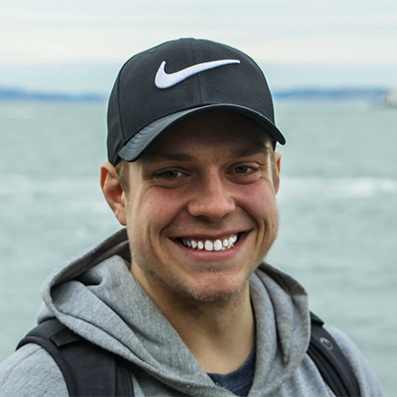
Creating a custom Sentry block using Prefect 2
In my last blog post, I described a practical way of integrating Sentry with Prefect 1 flows. Since then, Prefect 2 was released, and with it, many interesting capabilities were introduced. I won’t spend time explaining why you should migrate to Prefect 2, but I suggest you have a look at the new features presented here. If you’re interested, I also created a step by step GitHub repository showcasing some of the new features available so that you can try it yourself.
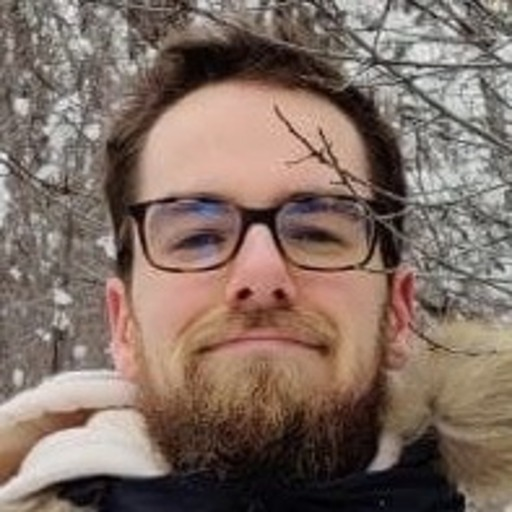
Coveo auto-reply integration with Slack
Use case
Following the newly launched Coveo Slack Application, it is now possible to search your whole Coveo index directly from Slack with a single click or slash command. This is definitely a great approach to search, but what about Slack users that are not aware of this feature but actually need it? This is the use-case we will tackle in this project: automatically answering questions in targeted Slack channels.
It is not a secret that some Slack channels become a search dump and it is not always easy to address them all, even when the answers are sometimes easy to find. A great way to tackle this problem is to try to find questions and send them to the Coveo Search API. A simple way to do this is to listen to all the messages sent on a targeted channels, and only trigger a search on the ones containing a question mark.
A simple architecture for this idea would be to host a serverless function in AWS Lambda, and send the detected question to Coveo’s Search API. For better versioning, deployment, and monitoring approach, a serverless Application will be used for this project.
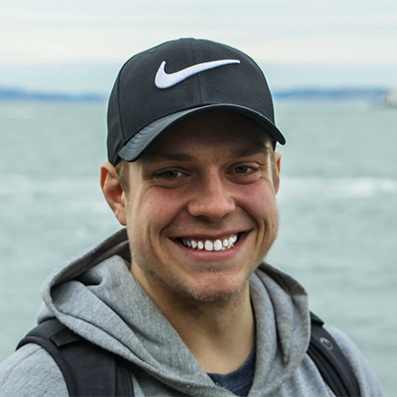
Integrating Sentry in Prefect Flows
At Coveo, we deal with an enormous amount of data on a daily basis. With data growth,
our data platform has also grown from a single team a few years back, to more than 3 teams and 20 employees.
With this growth, we also gave ourselves the mission of democratizing data across our organization
and allowing more and more external teams to access and experiment with the data we capture.
The challenge we rapidly faced was that we had to offer more and more support to these external teams on how to
automate some of these applications and scripts they were developing over the data. Most of these stakeholders are
often really proficient with SQL and Python, but have less knowledge and experience with CI/CD,
infrastructure, and monitoring.
To solve this problem, we started looking at some solutions that would allow these teams and individuals to easily
deploy and run these different workloads in production, without having to develop an in-house solution that would
require a lot of engineering time and maintenance.
After investigating multiple solutions to solve this problem, a clear winner stood out for us: Prefect.
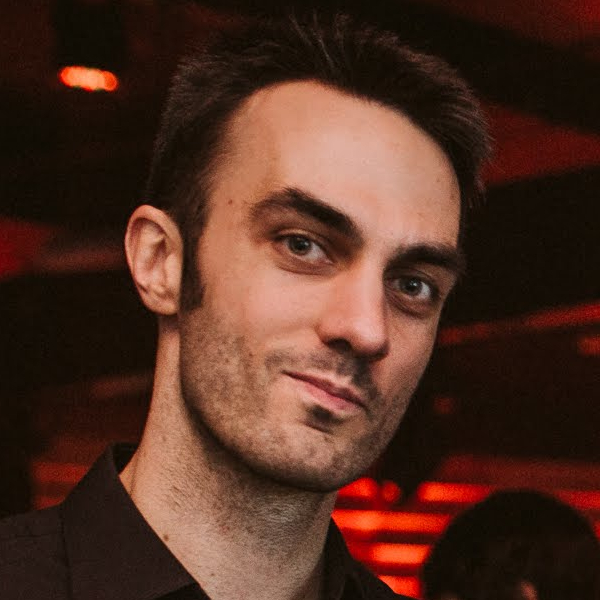
Software Engineering: It’s All About Abstraction
Next summer, I’m going to drink a beer under a tree.
Right now you probably have an image of me, under a tree, with a beer: you understood my intent.
What’s interesting is that the details of this image will probably be significantly different for each person that is reading.
This is because I did not talk about the implementation; beer and tree are abstractions.
They efficiently convey a general idea without getting lost in the details.
Efficiently is the key word here; imagine if I had to describe the detailed implementation of a tree or a beer each time I want to talk about them! 😰
Since abstractions are a key part of the way humans communicate, they are also a key part of software engineering.
Indeed, since software engineering is about programming over time, it is in part about using source code to communicate ideas to other developers.
This won’t come as a surprise to most of you; we, developers, know that abstractions are a key part of our job.
That being said, it’s not always clear how abstractions link to our day-to-day programming and to our leading practices.
Single Responsibility Principle (SRP), Design Patterns, Don’t Repeat Yourself (DRY), and test readability are all somehow related to abstractions.
This post explores these connections and shows how understanding them helps to make better decisions about our programs.
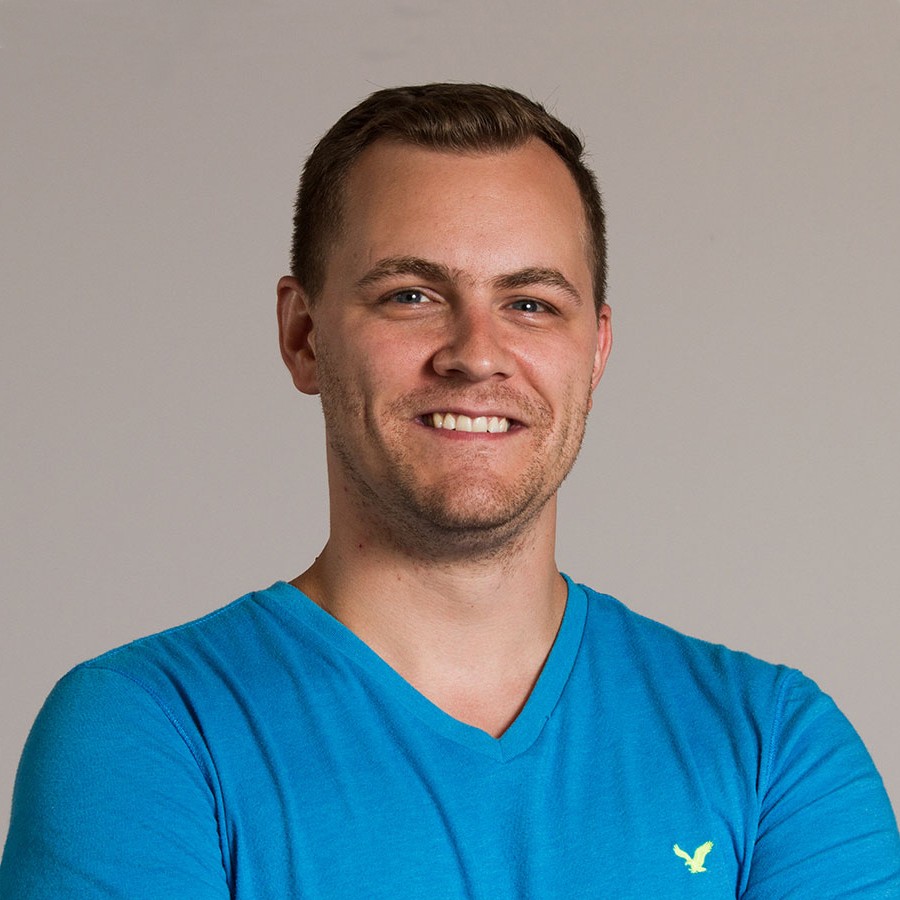
Using Amazon Aurora Global Databases With Spring
A few years ago, Coveo wanted to offer the possibility to store customer data outside the United States. Early in the project, it was decided that we would only have one user directory. If our users had to manage organizations created in different regions, we wanted them to avoid having to log in each region individually. This meant that we would have only one database to store users. This blog post will explain how we achieved a central user directory while avoiding big latency issues.
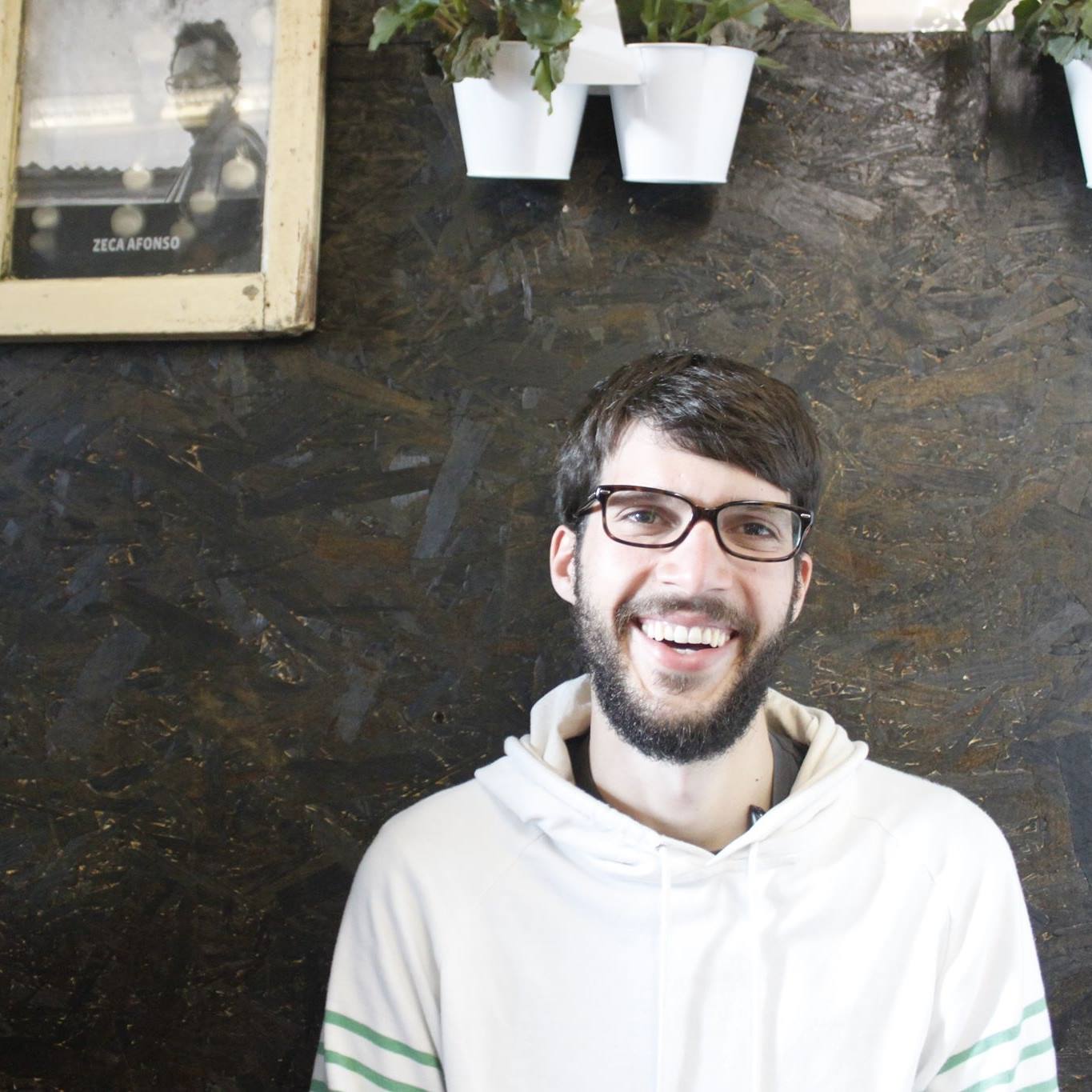
Leveling in code review
Through the years, I’ve heard many different opinions about code review, ranging from “publishing code to the main branch with extra annoying steps” to “I learn so much from it” stepping through “it’s pair programming with delay” and “I can’t push to prod unless someone looks at my stuff”. Being a big fan of code review, I’d like to share how I make the most of it and how I can learn from people who are awesome at it and ideally help you get to a place where you can provide a lot of value with it.
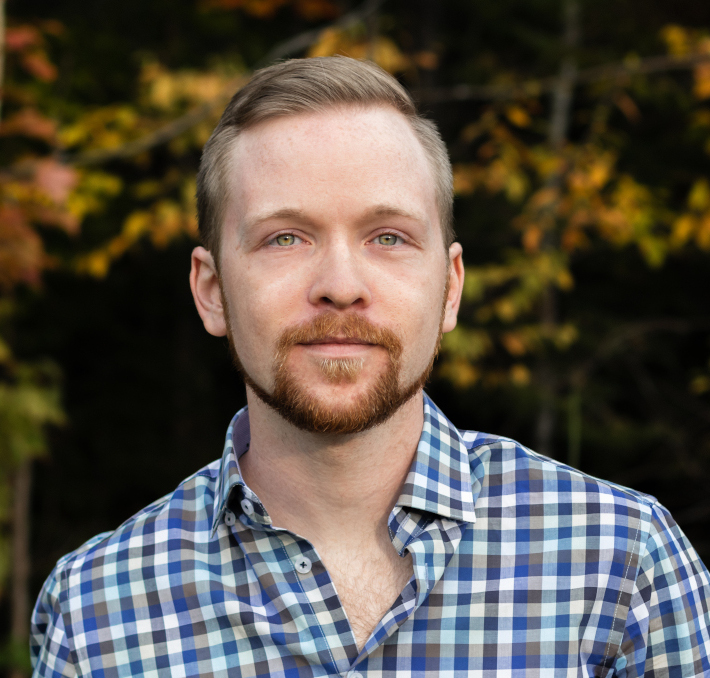
Prometheus at scale
Coveo has experienced great growth over the last few years, by bringing in new clients, deploying in new regions, integrating new technologies, etc. But the infrastructure on which our offering sits must follow the same trend. This explosion in data and event volumes demands that companies find scalable solutions to match their ambitions. It’s a bit more complicated than simply throwing buzzwords like incantations, so I’ll help you dive a bit into this world.
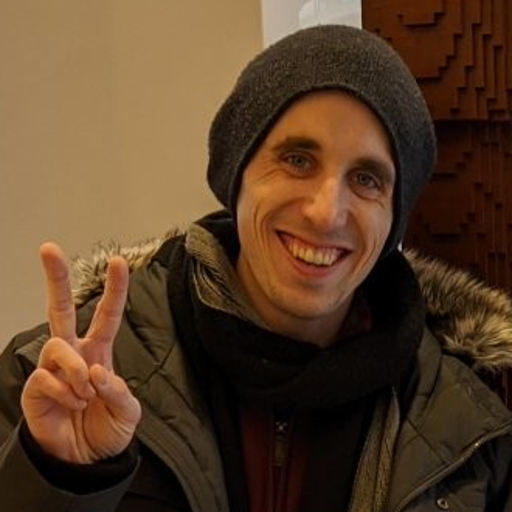
Query Suggest and Multi-Threading
Coveo’s Query Suggest model provides highly relevant and personalized suggestions as users type. In this blog post, I will explain how Query Suggest works in the back end, and how it uses mutli-threading to provide results at high speed.